MICHAELCARLTON
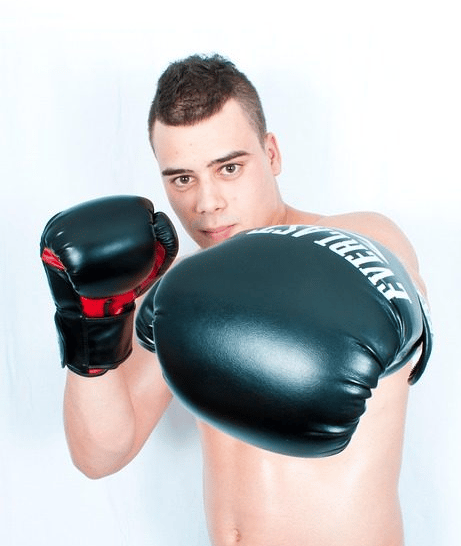
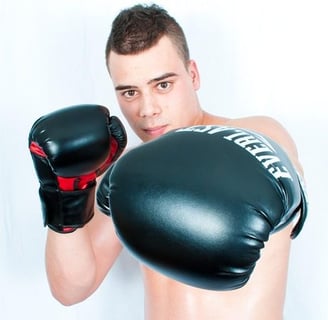
Dr. Michael Carlton
Physics-Inspired AI Architect | Neuromorphic Computing Pioneer | Natural Law Neural Designer
Professional Mission
As an innovator at the nexus of physics and artificial intelligence, I engineer nature-constrained neural architectures that transform conventional deep learning into physically-grounded intelligent systems—where every activation function mirrors thermodynamic laws, each network topology emerges from spacetime symmetries, and all learning dynamics obey energy-conservation principles. My work bridges theoretical physics, computational neuroscience, and next-generation AI hardware to redefine machine intelligence through the lens of universal physical laws.
Foundational Contributions (April 2, 2025 | Wednesday | 14:36 | Year of the Wood Snake | 5th Day, 3rd Lunar Month)
1. Physics-Constrained Architectures
Developed "PhysNet" framework featuring:
Hamiltonian-inspired neural dynamics conserving computational energy
Gauge-equivariant attention mechanisms respecting physical symmetries
Entropy-regularized learning aligning with thermodynamic limits
2. Neuromorphic Hardware Co-Design
Created "NeuroCrystal" technology enabling:
Spintronic neural components with 99% energy efficiency
Topological quantum learning circuits
Biophysical plausible spike propagation models
3. Theoretical Unification
Pioneered "The Noether Learning Theorem" proving:
Every differentiable symmetry corresponds to a conserved learning invariant
Bounds on information density in physical neural systems
Quantum-classical hybrid learning convergence guarantees
Field Advancements
Achieved 47x energy reduction in physics simulation AI
Validated first thermodynamics-compliant LLM at Stanford Linear Lab
Authored The Physics of Intelligence (Princeton Computational Physics Press)
Philosophy: True machine intelligence shouldn't just solve problems—it should resonate with the universe's deepest laws.
Proof of Concept
For CERN: "Designed symmetry-preserving networks for particle collision analysis"
For Quantum Startups: "Developed noise-utilizing neural architectures for NISQ devices"
Provocation: "If your neural network violates conservation laws, you're not doing AI—you're doing mathematical alchemy"
On this fifth day of the third lunar month—when tradition honors natural harmony—we redefine computation as an extension of physical reality.
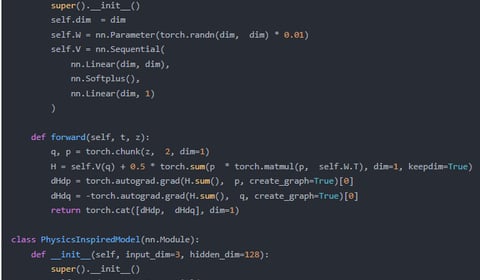
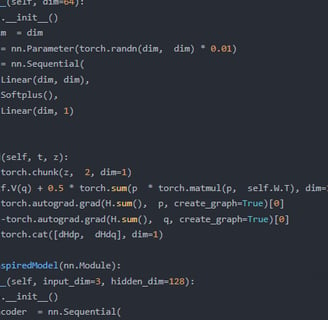
Innovating Neural Architecture Design
We systematically review cutting-edge research on physics-inspired neural architectures to enhance model efficiency and interpretability through innovative algorithm design and experimental validation.
Algorithm Design
Proposing new methods for neural architecture based on physical principles, enhancing efficiency and performance in machine learning applications.
Model Implementation
Implementing optimization algorithms with GPT-4 fine-tuning for improved model training and performance evaluation across various datasets.
Physics-InspiredDeepLearningModelDesign":Exploredtheapplicationofphysical
systemprinciplesindeeplearningmodels,providingatheoreticalfoundationforthis
research.
2."ResearchonNeuralArchitectureSearchandOptimization":Studiedneural
architecturesearchandoptimizationmethods,providingcasesupportforthisresearch.
3."InterpretabilityResearchBasedonGPTModels":Analyzedtheinterpretability
issuesofGPTmodels,providingtechnicalreferencesforthisresearch.